Expected Goals (xG): The Influence of Data in Football Betting
Data is one of the big buzzwords in football these days. We are constantly hearing stories about how big clubs are successful because of their use of use of data, but it also enables smaller, but smarter, clubs like Brentford in the Premier League to fight way above their weight class by utilizing it correctly.
However, it is not only the clubs that can take advantage of data; it is also a useful tool for smart bettors. Here, we are naturally not speaking about obvious data such as goals scored or matches won, but underlying performance metrics, which is better at predicting future outcomes.
Terms such as expected goals, better known as xG, expected points, xP and the Table of Justice have become household expressions within the football vocabulary. But how do you use them to increase your betting winnings?
Data and statistics in football
Since the betting companies and professional clubs starting early adopted the use of xG, the expected number of goals has become an often referred to data point.
xG has gone from being a metric in the spreadsheets of the data analysts to being regularly mentioned by both pundits and managers in the Premier League these days.
We are also seeing it frequently mentioned within fantasy football, where a players xG or expected assists, xA, are often referred to when gamers attempt to decide which players will score the most points in the next round.
Expected goals: What Is xG?
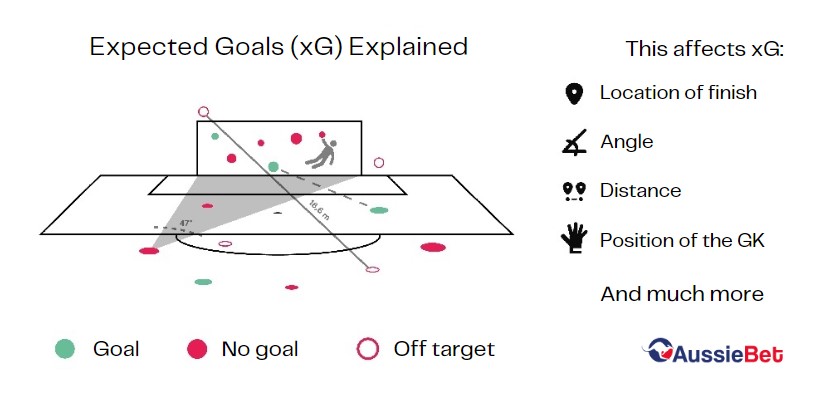
Simply put, xG tells us the likelihood of any given shot’s chance of turning into a goal. This is based on the context surrounding the shot, and every shot is given a number between zero and one. The closer to one, the bigger the chance is.
Every single shot is compared with thousands of previous shots with similar context to determine the likelihood of the shot turning into a goal. This is stuff like the distance, the angle of the shot, the positioning of the goalkeeper, the number of defenders between the ball and goal, which foot the striker is using and much more.
The xG value is thus revealing more about a team’s performances, the strength of their defence and offence and even the individual players than what the actual match result or some other metrics show.
xG is like a miniskirt
There are some old school football fans who sees this kind of data as useless and as a modern and nerdy way of looking at the beautiful game. And while it is true that there are some weaknesses to being too xG-centric, there are also a lot of advantages to the metric.
To quote legendary Danish football coach Ebbe Skovdahl: “Statistics are like a miniskirt. They give a lot of good ideas but hides what is most important.” The same can be said about xG, which is useless unless put into context and analysed properly.
The xG methodology was developed to help clubs understand better why they were winning and losing matches, and what they could do to optimize performances going forward.
Previously, the most cited metrics were ball possession, which coaches cared about since they figured their teams had a higher chance of scoring and lower chance of conceding goals if they had the ball a lot.
Later, shots, especially those on target, an important factor as more shots meant higher chance of scoring. However, not all shots, on target or not, are created equal. This is where xG enters the scene.
Let’s imagine a match between Team A and Team B. Team A has five shots and team B has ten. On paper, it seems like Team B has performed the best here. However, if we then look at the shot chart, it shows that all of Team B’s shots came from outside of the penalty box, while Team A’s five shots came close to the six-yard box. This means that Team A had the best chances and deserved to score most goals and win the match.
All football fans have at some point experienced watching their team create multiple big chances in a match only for the opponent to score a last-minute goal on a long shot. Afterwards, they have told anyone that would listen how the opponent was lucky and didn’t deserve the victory because their team should have scored at least four goals. With the xG data, they can prove that this is the case.
How xG is calculated
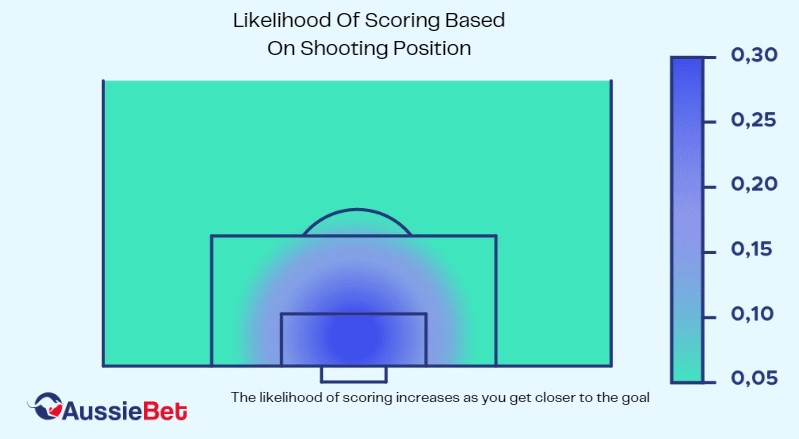
The big remaining question is obviously how this xG number is calculated. It is done through machine learning models based on hundreds of thousands of shots.. With every single finish from thousands of football matches at top level each season, the data becomes better and better.
On top of this, one must add the beforementioned variables, such as the goalkeeper’s position and defenders, that influences the likelihood of a goal to be scored.
Most football fans can determine whether a shot has a big or small chance of ending in a goal. However, even the biggest football fans won’t be able to see enough shots over a match, not even a full season, to use this data to determine the scoring chance of these unique situations. This is why we need the xG models as they include more shots than even the most hardcore fans have ever watched in TV or the stadiums.
The xG model saw the light of day at the start of this century, and today there are multiple models that calculate the likelihood of goals.
The differences are mostly which variables that are taken into consideration. Generally, it can be said that the most accurate models are those that includes more information and variables, while the less accurate ones are the simple ones. It can also be added, that the xG data you get for free on most livescore sites is generally significantly less accurate than the data clubs and professional betting syndicates buy and work with.
A cheap xG model might for example only take the distance to the goal, the angle of the shot, the body part and type of assist into consideration before concluding an xG of 0.45. A more precise model from for example the data company Opta, will also add the goalkeeper’s position and status, the positions of all the strikers and defenders and whether the shot was taken with the striker’s weak or strong food into the equation.
If you know that the keeper was out of position, the same finish as before, could have an xG of 0.65 instead. Alternatively, it could also be an xG of 0.20 if there are seven defenders between the ball and the goal.
Pros and Cons of xG
The biggest advantage of xG is that it tells us more about the match than the result. It does so by telling who deserved to win based on the chances created. Furthermore, xG can help us analyse player performances in depth by eliminating luck from the equation.
On the other hand, cynics will point out that actual goals in actual matches matters more than these calculations. And furthermore, that xG truthers tend to overestimate the value of the metric. The fact that Team A creates an xG of 2.00 and Team B only 1.00 doesn’t necessarily mean that Team A was the best team and deserved to win 2-1. It means, in best case, that Team A created more chances of higher quality and had a higher chance of winning.
But there is also the case that 1.95 of Team A’s chances could have been created in the last two minutes of the match, and that Team B had been dominating completely for the first 88 minutes.
Another weakness is that xG doesn’t take the players’ abilities into consideration. A skilled striker and a central defender will both get the same xG if they take the same shot. This also means that finishing ability and the level of the goalkeeper isn’t part of the equation.
xG also only count shots. Dangerous attacks or situations where a shot isn’t taken is not being measured. This means that if a striker is one on one with the goalkeeper, and tries to dribble past him unsuccessfully, it won’t be added to the xG tally despite the chance being huge.
You can not use xG to say anything about the match picture either. You will often see a team go ahead in the first half and then decide to play more defensively afterwards to protect the lead. This often means that the opponent will accumulate a higher xG as they take more shots because they are forced forward. At the end of the game, it might look like they deserved the victory even though it was because they fell behind that they had to shoot more.
Generally, the xG of teams leading for a long time, will look worse than those of teams that are behind. This is because teams leading play more cautiously and fall back, which then gives away more shooting opportunities to the opponent and less for themselves.
This is where the context is once again a crucial element of the xG analysis. It is important to not only look at the total accumulative xG, but also to take the quality of each shot into consideration. A penalty has an xG of 0.78 and can thus skew the data significantly. Often, one should remove penalties from the total xG.
If a team creates a total xG of 2.20, but gets two penalties, they only create 0.42 xG outside of the penalties in the entire match, which isn’t a lot. In that case the opponent were likely to have created much more, had it not been for the penalties.
Furthermore, we must return to the example from the beginning. Two teams can both end up with an xG of 1.5, but they can be created differently. One team could get the xG after taking 20 long shots in the match, while the other has three big chances worth 0.5 xG each.
Even though both teams would have the same xG, the second team with the few, but big, chances have a much higher chance of scoring (multiple) goals. It is better to create bigger chances, even if you don’t get as many of them, than to take a ton of shots from the distance.
This is one of the reasons for why the better and more data driven teams these days take less shots from distance and favour trying to get through for big chances.
xPoints and Table of Justice
Most football fans these days have heard clubs refer to the Table of Justice. This is often coming from coaches of underperforming teams, who are trying to explain that their team is performing better than what the results and standings show.
The Table of Justice is built upon the xG data through what is known as expected points, xPoints.
The xPoints is calculated by looking at how many points each team should have gotten based on their accumulative xG in their matches played. This is done with a Monte Carlo-simulation where we can calculate the outcome of a situation through multiple random simulations.
Another, and simpler way, is to use the xG differences from the matches and give a point total based on the difference. Because of the reasons explained above about the context of matches there are some weaknesses to this approach, but spread over a full season, these are minimized at least.
xG difference | xPoints |
1.5+ | 2.7 |
1.5<1.0 | 2.3 |
1.0<0.5 | 2.0 |
0.5<0.0 | 1.5 |
0.0<-0.5 | 0.7 |
-0.5<-1.0 | 0.3 |
<-1.5 | 0.1 |
xPoints can thus be compared with the actual number of points won by the team. If the difference between the two is big, this is something to examine further.
It could either show a team overperforming and collecting more points than they should. If this is the case, one should prepare to bet against them as lucky streaks never last, and you can get a higher odds on the luck running out.
The opposite could also be the case with a team underperforming and getting less points than they deserve. In that case, you want to bet on them to win their next match as luck should turn around the corner.
What Can the Values be Used for?
Since xG helps us look further than just the results and get a better understanding of the quality of teams and players, xG can also be used in betting.
With xG it becomes possible to find differences between what should have happened and what actually happened, which is useful when trying to predict what happens in the future. As stated earlier, a team that are performing better or worse compared to their xG, will most likely regress to their norm sooner or later.
In recent years, the bookmakers have started using xG more and more when setting their odds. This has made it harder to fine value bets through xG alone, but it remains an important factor to take into consideration before placing a bet.
Team Analysis
xG and xG against (xGA) gives us more accurate pictures of the quality of chances created or allowed. This is highly valuable in a sport like football that doesn’t have a lot of goals.
To take an example, we can use the xG to show which teams create the most quality chances. In the Premier League, it is no surprise that Manchester City and Liverpool are the best performing teams on this parameter.
In the 2023/2024-season, Liverpool had an xG of 94.79 and scored 86 goals. Meanwhile, Manchester City had an xG of “just” 89.55 but they scored 96 goals. These numbers show us that Liverpool created better chances, but that Manchester City were significantly better finishers as they overperformed their expected goals.
In the other end of the scale, Everton were the poorest performing team as they scored just 40 goals on an xG of 60.75. Their finishing was thus terrible as they scored almost 21 goals less than they should have.
Player Analysis
xG can also be used to measure the production of players in the offensive part of the game. It is naturally limited to only the quality of shots and chances created by a player, but for bettors it remains useful. This is for example when betting on player props such as anytime goalscorer, anytime assist or shots on goal in a match.
The xG data can also be used to analyse goalkeepers. Just like it is a good thing when a striker scores more goals than their xG indicates they should, it is great when a goalkeeper concedes fewer goals than what the data suggests he should. When evaluating goalkeepers, we only include the shots on target of course since shots wide or over are irrelevant. This is called xGOT – expected goals on target.
If you want to bet on anytime goalscorer market, it is therefore smart to look at how the opposing goalkeeper is doing. And then you should look at the quality of the chances the player is normally getting. Here, you can also look at whether the player is finishing well or not.
Conclusion
It is important to keep in mind that all xG data is based on models and estimations. It is a mistake to think that xG tells the full story of a match. In best case, the xG data presents one story of a match.
Different models will give different results, and it is important to remember this when analysing a match.
The average of the different models is likely to give a better overview than if you use only one source. Even though the xG data is a very useful source of information, they are furthermore best utilized in collaboration with other data and context when trying to find value bets.
xG is still a tool under development and it will only grow more and more accurate as more data becomes available. It is important not to ignore the surrounding context and also look at the playing style and individual player quality when using xG as a tool in betting.